Artificial intelligence and machine learning have become key applications of technology with their presence being felt across different sectors of human activity. However, this rise to prominence has not been without problems. Certain key issues have become more apparent since humanity’s enhanced efforts to study and deploy AI for its ends. Among these is an issue that has worked to dampen human trust in artificial intelligence, essentially calling for reevaluations and assessments about these algorithms’ fidelity. AI bias, as we know it, has become a central point of contention, with several critics of AI technologies pointing out this pitfall as a major flaw in the processes of functional algorithms. However, this problem finds its roots in the creation of AI and ML algorithms in themselves, rather than being solely an operational conundrum. Artificial intelligence invariably relies on datasets and a stored volume of training information. Limitations of these datasets often restrict the protocol’s ability to decipher and handle tasks that may lie outside the scope of these information blocks.
That being said, AI bias has become a key issue most major AI firms are trying to actively address, with OpenAI, Google, and Anthropic repeatedly reaffirming their commitment to responsible AI and reducing the instances of hallucination in their respective chatbots. While these firms have been successful in mitigating the instances of bias and problematic responses, they haven’t eliminated the dubiety in AI. This brings us to the question of whether AI bias can ever be addressed comprehensively. The upcoming portions of this article discuss this matter in greater depth.
What Makes AI Bias Significant?
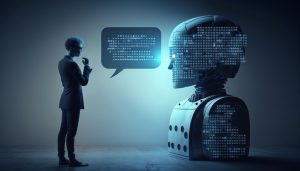
AI bias is capable of damaging human trust in artificial intelligence.
AI bias, essentially, is an extension of the biases ingrained in the data set the algorithm is trained on. To understand this phenomenon, it is important to decipher how bias creeps into the system. All AI algorithms are trained on vast blocks of information drawn from the internet. A significant portion of these information blocks comprise data taken from interactions of people on social media platforms and other forums. Humans, as we know it, are prone to several biases based on their social conditioning, education, and levels of exposure to the world around them. Needless to say, generative AI algorithms often end up replicating these biases, albeit in a strangely different manner. While AI bias examples can include offensive responses regarding complex sociological concepts such as sex, race, or gender, the phenomenon can also entail a simplistic omission of a particular form of a response merely because the underlying language model didn’t contain related data. While AI bias has indeed come to bear rather ominous connotations, technically, it remains a problem that can be mitigated using balanced data sets and information.
However, the question surrounding the elimination of AI bias still remains. While it might not be possible to remove bias from AI systems entirely, it is certainly feasible to understand and reduce the instances of bias within an algorithm and its responses. As chatbots like ChatGPT, Bard, and Claude gain popularity, people must understand that despite these tools having access to a vast database of information, they’re still prone to errors and pitfalls. While researchers work to build better neural networks and enhance the capabilities of extant deep learning mechanisms, it is equally essential to navigate the pathways of AI bias to prevent major mishaps from AI-supported systems. With artificial intelligence having made it to key positions in the decision-making framework for some processes such as cybersecurity and finance, it becomes crucial to minimize the occurrence of bias to ensure faulty conclusions do not turn into larger cascades.
Managing Bias and Its Consequences in Generative AI
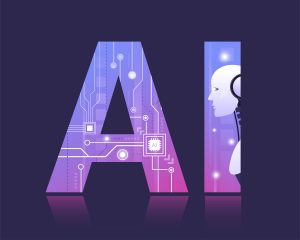
Complete elimination of AI bias might not be possible.
The first step in addressing AI bias is to look for any potential gaps in the training data. Most commonly, deficiencies in the information present in these databases are often the result of biased or incorrect responses. The absence of this information prompts the AI to often extrapolate based on its training information, leading to faulty or inaccurate responses. This can be managed by including a broader scale of information along with including variety, with the insertion of numerous different variants of a similar form of information within the dataset. As AI moves to assist with medical technologies and other crucial aids in high-stakes industries, it’s important to provide language models with sufficient information of all sorts to prevent incorrect conclusions. AI development firms might also have to evaluate existing processes at their firms to weed out any systemic biases or attitudes that might be percolating down to the final product. Proactive measures in addressing these concerns will also aid in minimizing bias from the resulting chatbot.
Auditing and reviewing AI responses from time to time also help refine the overall quality of AI, aiding the algorithm to prevent harmful or spurious responses. Firms like Inflection.ai have developed unique mechanisms to aid approachability and friendliness in AI by focusing on minimizing the extent of potentially questionable responses and bias. Anthropic, too, is consistently working on similar paradigms, having achieved more success with its latest chatbot—Claude 2. However, it’s important to note that any algorithm based on natural language processing or otherwise will ultimately be predisposed to some degree of bias since the main sources of these biases are their human developers. Despite minimization, a trace of AI bias will remain, which essentially calls upon human users to always rely on their faculties of critical thinking and intuitive cognition despite using AI for some of their tasks.
How Data Bias in AI Will Shape the Industry’s Trajectory
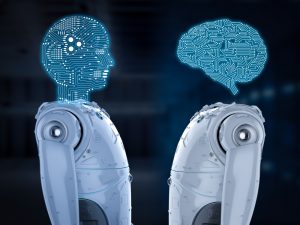
AI bias is among the major concerns and pitfalls of modern AI algorithms.
As time progresses humans will invariably unlock more pathways to learn about as well as develop better AI protocols. That being said, AI bias continues to remain a factor in the proceedings concerning the development as well as usage of artificial intelligence. Enhancing transparency remains a goal of most firms invested in AI development, as regulations on artificial intelligence continue to grow and bring more statutes under their ambit. More organically, however, AI developers might also have to spend some time understanding how bias in humans works to better estimate its impact on language models and other machine learning algorithms. The process remains ongoing, given that humans are still in the initial stages of advanced AI development.
FAQs
1. Can bias be removed from AI?
While bias can be managed and mitigated using better quality data, complete removal of AI bias seems unlikely. Integrating checks and balances to minimize bias will remain integral to suppressing overt and covert biases in artificial intelligence.
2. Why is AI bias complex?
Since AI algorithms are created by vast teams of professionals, conscious and subconscious biases might creep into the process. Moreover, AI relies on data crawled from the internet to support its responses and decision-making. These datasets might often contain opinions and biased information leading to bias creeping within the resultant AI protocols.
3. Is AI always biased?
Not all responses from an AI are biased. Bias often results from ignorance of a specific piece of information that ends up shaping the protocol’s response to a query. This often leads to outputs that are deficient in information or outright absurd since AI models tend to hallucinate.