Banking is among the most high-stakes domains in the modern economy. These institutions are important not just for economic stability but also to promote better wealth management and cash flows. The advent of language model artificial intelligence and natural language processing has touched just about every industry and continues to open up new opportunities in streamlining existing processes. This applies to the banking sector just as much as other financial services. While blockchain and the associated AI component are already carving out a good chunk of the alternative finance industry, AI for banking will propel existing institutions into a new approach in the upcoming decades. Disciplines of artificial intelligence such as analytics and big data can be of great use to financial institutions that generate a vast volume of sensitive information. Apart from these critical aspects, AI banking can also enhance the extant customer service section of financial services, ensuring customer satisfaction and ease.
The progressive inclusion of AI tools and technologies in cybersecurity and encryption has also aided better security measures in what is a highly digitized banking market. Extensive usage of digital systems has also necessitated capable security measures. While human intervention and critical thinking are absolute in these aspects, the inclusion of automated AI tools and intelligent algorithms will further enhance the robustness of financial security protocols. Moreover, prevention of financial crimes and early detection of untoward activity can also be made possible. The upcoming sections explore the potential of AI in banking, its implications, and challenges.
Banking AI: What It Entails
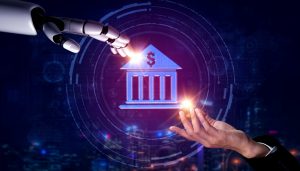
AI in banking can provide intuitive solutions to several conventional as well as high-risk processes.
Advanced artificial intelligence and machine learning algorithms have progressively made several processes easier in the banking and financial industry. Among the simplest use cases for the deployment of AI is automated customer service chatbots. Banks need effective systems in place to address a high volume of customer calls and queries. AI can simplify and streamline the process by managing several customer contacts. Consistent advancement in chatbots has made the outreach experience straightforward and more intuitive, directing customers to the exact departments they need to contact. Human-like natural language flow and intuitive conversational mechanics also aid with customer outreach and can help banks with generic customer inquiries by providing basic information. This could include account application processes, interest rates, and the bank’s terms of service. In more advanced use cases, artificial intelligence can help mitigate financial risk and help point out suspicious activity and patterns, even if posed with gargantuan amounts of transactional data. The proliferation of AI in law and legal processes can bring synergy between banking systems and existing regulations.
Recent advances such as the Advanced Data Analysis plugin in ChatGPT have furthered the prospects of maximizing data science penetration in everyday lives. Banks can now come up with more affordable AI solutions to address key tasks such as wealth management and asset allocation. The same technology can also promote automated credit risk evaluation, which today requires several metrics drawn from a broad spectrum of information. More importantly, AI can also help banks make better decisions as strategies and techniques surrounding AI protocols in business continue to grow steadily. The more sensitive use cases apart, AI’s tendency to automate mundane tasks also allows financial institutions to seamlessly create marketing and advertising campaigns, now that AI image generation and video creation platforms are widely available.
The Challenges of Using AI for Banking
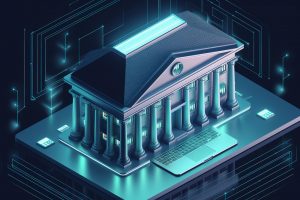
AI still holds several challenges and caveats. These will have to be addressed prior to the adoption of AI in banking.
While AI and ML have certainly become far more popular than they ever were before, several organizations are still coming to terms with the diverse prospects of integrating these systems into their existing frameworks. Progress in this regard is varied, and each bank has its own set of challenges. Adopting AI comes with a fair degree of risk and also needs human operators that keep a close eye on the algorithms. Apart from added cost, the prevalence of phenomena such as AI bias and hallucination further complicate the prospects of utilizing artificial intelligence in high-risk aspects of commercial banking. Banks using data analytics also need to ensure the quality of data they generate is top-notch and accounted for. Poor data quality impacts AI’s performance and can hamper the solutions it provides.
Other challenges include banking operators that still use dated and legacy systems in their frameworks. Such organizations will find it difficult to integrate AI into banking processes. Modern, cutting-edge banking technology is a more recent phenomenon. AI in financial services is just being considered and popularized, now that several professionals are noticing the potential it can bring to the markets. The competition and rivalry wrought by major tech companies in the language model space have certainly contributed to this phenomenon, but more banking-specific AI development will be required to effectively provide solutions to these institutions. Lastly, regulations surrounding the use of AI and ML in banking and other financial domains are not entirely clear. Unless these statutes are ratified and established, banks might be unwilling to take a risk and deploy protocols with unestablished regulatory approval.
The Outlook for AI Banking
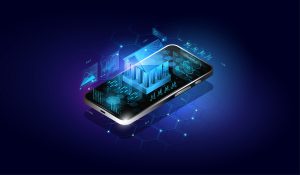
Integrating AI in banking is promising regardless of the challenges.
Despite the challenges, the outlook for AI banking seems bright and full of potential. As the markets remain conducive to consistent developments in artificial intelligence, the gradual percolation of these algorithms in banking processes is inevitable. While several banks already deploy ML protocols in specific processes, the greater usage of AI systems will only boost banking efficiency. That being said, there remain several unresolved challenges that will need to be progressively dealt with. The necessity of responsible AI development will grow by leaps and bounds since banking remains one of the most crucial legs of the economy and also the core wealth management system in society. AI ethics and regulatory stringency cannot be ignored for the sake of including modern technologies unless they’re up to the safety and privacy standards of financial institutions.
FAQs
1. How is AI used in banking?
AI can be deployed to manage customer service, analyze data, prevent financial risk, detect fraudulent activity, and enforce robust cybersecurity methods in banking.
2. What is the future of AI in banking?
The future of AI in banking looks promising. Recent advances in language models have renewed interest in AI development, promoting the spread of AI technologies to various domains. Banks require large-scale analytical, customer support, and security algorithms, which AI can seamlessly cater to.
3. What are the challenges of AI banking?
Despite being productive, AI still has pitfalls that can threaten key processes. Bias and hallucinations in AI will have to be mitigated if automated algorithms have to be integrated with sensitive banking processes. Regulatory nebulousness and limited knowledge also pose significant risks to the complete integration of AI in banking.