The growth of computing power and the complexity of neural networks has pushed forth a revolution in the field of information technology and in the development of machine learning. The past year has been especially eventful in showcasing the many wonders of machine learning and artificial intelligence with the development and release of numerous chatbots like ChatGPT. Schools, universities, and policymakers have been closely monitoring the effects of machine learning in education in the immediate aftermath of the launch of these language models. Therefore, projecting the future of machine learning for education has become a key topic of discussion. Along with machine learning comes analytics—a key component of the discipline—which has the potential to help teachers and educators make sense of large-scale student performance data while providing intuitive approaches to optimize student outcomes. Educational analytics can also pave the way for adaptive learning and personalized education for students, allowing them to learn on their own terms while remaining focused on key areas that require improvement.
Machine learning in education can also be helpful in administrative tasks, helping both students and teachers keep track of their records and also gaining access to them in a hassle-free manner. Apart from these obvious use cases for machine learning and analytics in education, there also exist numerous other opportunities for the integration of intelligent systems into the overarching educational framework present in current systems. With the advancement of the world around these institutions, the problems they face are also bound to become more convoluted. While machine learning might just help them address these concerns, a neutral assessment of this possibility is integral to understanding the impact of ML systems on academics at large.
Machine Learning in Education: Initial Impressions
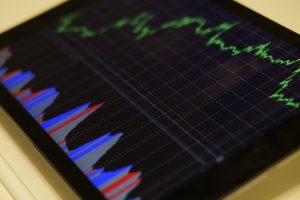
ML algorithms can help universities decode vast chunks of data and make sense of it.
Machine learning and analytics in education might have the potential to aid institutions to help students identify key areas that require improvement and optimize their efforts for success. These systems can first be used to generate data from existing performance records and other student-related metrics in an institution and then be analyzed later based on the various requirements. Students at risk of falling to mental stressors and other educational pressures can be identified through grades and rates of attendance. In such cases, analytics can help universities get to students that are at greater risk of performance-associated pressures and also those who might drop out. Following this, universities can come up with a plan for outreach and appropriate counseling supported by pointed help to retain these students and aid their improvement. While these use cases take into account only two primary metrics—attendance and performance—other crucial parameters such as financial stress and social well-being can also be added to the mix, allowing machine learning-aided systems to make sense of complex data with more variables.
Moreover, institutions can also look for metrics like creativity and intuitive excellence using defined parameters for the same. This might just allow them to find students that exceed conventional expectations. These methods of defining parameters and conducting analytics in education can transform the way universities understand their students. Most importantly, it allows them to craft highly specific tools to combat student issues while achieving high rates of engagement. The results of the corrective measures can also be studied, with data deciphered using higher education analytics to ensure the measures deployed are showing tangible results. With machine learning models, historical data is also made accessible with ease, helping university professionals to keep track of all programs initiated by the institution and their respective success rates. This will enable institutions to reflect on their measures and also improve upon what worked in the past while discarding the redundancies. These factors might also aid and transform the future of testing in education, all thanks to the specificity and accuracy provided by ML protocols. Apart from tracking student performance and risk, universities can also deploy machine learning and analytics to prime their marketing and advertising campaigns, helping them boost enrollment and expand their footprint among prospective students.
Deploying Machine Learning and Analytics in Education
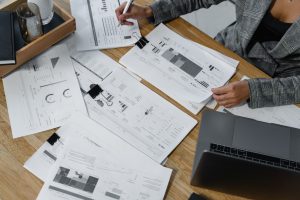
Insights from student analytics can help universities make changes to existing programs and optimize outcomes.
While both advanced computing solutions have a great deal of potential and advantages for institutions, they do require trained professionals and overseers to remain truly beneficial to universities. Apart from the costs associated with running these studies and analyses, universities might have to take a step-wise approach to implementing machine learning solutions in their institutions. Firstly, analyzing and defining the ideal student would be critical before undertaking a pointed study to improve student performance metrics. Most machine learning algorithms must be supported with the necessary patterns and parameters that need to be identified for them to work efficiently. The elimination of bias is equally important, and supporting the machine learning model with sufficiently large datasets with a range of variables is integral for accurate functioning. Carrying out test runs and comparing the results with existing factors and verified data can weed out any issues in the algorithms before deploying the model toward targeted goals in the institutions.
Higher education analytics can then help universities discover new insights and incorporate these findings into existing student support programs that might be targeted at various goals. Since machine learning models are still capable of producing biases and errors, institutions must also rely on the faculty of human intuition and training to inform their choices on the implementation of suggestions from the algorithm. Total and complete restructuring of existing methods is often not beneficial as it might require students to learn various new processes. This can often lead to detrimental results where an increased amount of time and resources are invested in return for minimal results. The suggestions from the algorithm must be assessed for various student groups and compared. The results might help universities inform other crucial augmentations to existing programs. Similarly, machine learning in education can also be deployed in novel concepts such as AI scoring and the revamping of student examinations.
Potential Limitations of Education Analytics
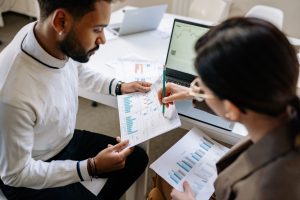
The extent of data sets often ends up limiting machine learning algorithms.
Despite the many intuitive solutions that machine learning-backed analytics can provide, technologies often come with limitations. Apart from the fact that language models and data analytics are restricted by the extent of their data sets, these technologies are also fairly expensive to implement. Also, the additional manpower required to institute these measures and alter existing systems based on the solutions provided by machine learning algorithms might command additional monetary resources. Since privacy and online security are of specific concern in the modern age, ensuring data security and the prevention of identity theft are equally important. Vulnerabilities in computing systems can often be exploited and result in data breaches. Moreover, the functioning of these solutions is highly specific and will require the identification of appropriate use cases before they’re acquisitioned and deployed. That being said, a balance of both human expertise and technical prowess will be crucial for these systems to become success stories in modern higher education.