Artificial intelligence has established itself as an everyday tool in human life with the massive traction garnered by generative AI in recent times. In its aftermath, several firms have consistently demonstrated their capabilities in creating commercially viable AI models for a variety of uses and applications. While still in its nascent stages, AI-generated content has demonstrated that it is capable of making a palpable impact on extant workflows in numerous fields. That being said, AI is not merely restricted to its generative capabilities and can be augmented to suit different use cases by altering its deep learning protocols. Among these potential AI applications is predictive analytics—a feature that has been popular among theorists and functional developers who have created predictive modeling applications using artificial intelligence. Predictive AI has been an area that is yet to be explored fully, given that its generative cousin has taken the front seat for the time being.
AI’s ability to spot patterns has always been at the core of its utility. While artificial intelligence might not be independently rational or possess critical thought, with sufficient training, AI algorithms can end up detecting patterns in data and raw information. Though generative AI does rely on a degree of predictive modeling whenever the algorithm attempts to create content in response to a user prompt, the front-end deployment of generative and predictive AI is starkly different. This article explores the differences between generative AI and predictive AI while also distinguishing their use cases and applications.
What is Predictive AI?
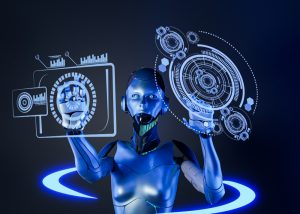
Predictive AI has numerous functional differences with generative AI applications.
Humanity has been generating vast amounts of data ever since the turn of the information age. The amount of data generated and stored has grown exponentially, alongside its consumption to aid informed decision-making. While humans are adept at noticing patterns themselves, manual data processing and interpretations take time and are invariably prone to human error. While AI, too, is not free of its biases, it can still be trained to look for very specific configurations within a block of data to detect similar features in broader volumes of information. Moreover, AI might also be more capable of making sense of structured data since machine learning protocols endow simultaneous processing capabilities to these algorithms. All businesses, government agencies, and non-profit groups function on some degree of historical information and work to predict the future by referring to past patterns. With predictive AI and analytics, humans can simplify the process of identifying significant bits of information, recognizing the pattern, as well as projecting potential outcomes and probabilities based on extant records. The use of computers and advanced neural networks to deploy predictive AI applications can greatly simplify and improve the decision-making process in data-reliant operations.
The functioning of predictive AI differs from generative AI in the fact that it does not always require an underlying language or visual model to function. Most predictive AI applications use highly specialized data sets that are curated to fit a very niche use case so that the machine can be adept at looking for those exact patterns. While this makes the machine highly specific, predictive training protocols can also make machines rigid, making it difficult to identify alternative data structures using the same model unless trained. Such instances might also make the model hallucinate, potentially creating issues with the predictive framework. Predictive AI models collect data and begin organizing the information into processable units. These units then undergo preprocessing to remove invalid or void information that might otherwise hinder the process of pattern recognition. Apart from these decisions, developers will have to select appropriate algorithms to support the machine learning model to aid with accurate results. Following these stages, predictive AI tools are often evaluated as benchmark tests to ascertain their accuracy.
What Sets Generative AI Apart from Predictive AI
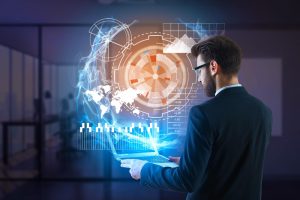
Both generative and predictive AI models have distinct use cases and are suited for varied applications within their niche.
Generative artificial intelligence is primarily concerned with the creation of content by deploying AI models. AI-generated content spans various domains including text, visual arts, videos, and even synthetic data. The most common use case for generative AI in recent times has been to support AI writing requirements alongside image generation protocols that have risen in popularity. Popular chatbots such as ChatGPT and Bard function using an underlying language model to support their generative functions. Image generation protocols like Dall-E and Midjourney also use language models and other AI algorithms such as generative adversarial networks to produce the best result. In essence, generative artificial intelligence is trained to create content similar to or related to the information present in the AI tool’s dataset.
Unlike generative AI, which uses existing information to create original but related content, predictive AI functions a tad bit differently. These AI protocols use deep learning, advanced statistical modeling algorithms, and niche machine learning setups to predict future patterns based on past and present information. Predictive AI can be deployed to predict the weather, calculate flight paths, make stock market predictions, and aid AI business applications. Predictive AI brings together the world of big data and advanced artificial intelligence to produce effective forecasting capabilities. Predictive modeling is also utilized in other AI applications such as speech recognition, speech-to-text conversions, and investment strategy creation.
The Scope for Predictive and Generative AI Applications
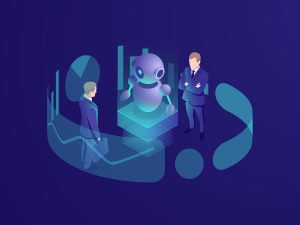
Predictive and generative AI applications are still in their infancy and have a great deal of potential.
With generative AI’s steady rise in recent times, the market for AI in general has witnessed a much-needed catalysis. This has triggered newfound interest in technology consumers to witness and use more AI products. Owing to prevalent market conditions, both generative and predictive AI applications have a bright future ahead, making way for innovation and development in the field. Numerous domains are already witnessing steady transformation with the arrival of AI, and enhanced access to these technologies is only set to further set forth new chains of events. While predictive AI can aid humans with data-driven analytics, generative artificial intelligence can help automate some of the more mundane aspects of content creation. Though neither of these can be independent decision-makers on their own, their support might just turn out to be invaluable in the coming years.
FAQs
1. What does predictive AI do?
Predictive AI is adept at identifying patterns in current and past data to accurately forecast future events. Predictive artificial intelligence can be used for a host of applications that rely on the accurate foretelling of future events. This includes meteorological predictions, financial market analysis, structural predictions, and environmental forecasting.
2. Does generative AI use predictive AI applications?
Generative AI and predictive AI are distinct. While generative AI might deploy some predictive features to create unique content, it is not adept at making forecasts about patterns or information contained within its dataset. Predictive AI, on the other hand, uses extant data to accurately predict future events and patterns.
3. What are examples of generative AI?
Popular chatbots like ChatGPT, Bard, and Claude and image generation platforms like Midjourney, Dall-E, and Stable Diffusion are all examples of generative artificial intelligence.