Natural language processing (NLP) has been one of the most talked-about technologies in recent times, thanks to the rapid development and expansion of AI chatbots and large language models. The discipline integrates subjects like computer science and advanced linguistics to allow machines to better understand human language. So far, NLP has been significant in bringing out a variety of facets that have been attributed to artificial intelligence. This includes functions such as AI writing and summarization, which have formed the bulwark of AI promotion following ChatGPT’s groundbreaking launch in the latter half of 2022. However, it would be unfair to say that natural language processing and AI have been without issue, for the truth seems to be the opposite.
Natural language processing, like all novel technologies, has its share of problems and outstanding concerns, which scientists and developers are still intent on addressing. While some of these are rather simplistic, others are complex and might even impact the future of NLP’s application in crucial algorithms and paradigms. Problems such as AI bias and hallucination are well-known issues; however, there exist other limitations that might be just as integral to address to ensure the long-term, sustainable growth of machine learning applications in human activities. The forthcoming sections will explore some of these issues in greater depth.
Exploring AI’s Disadvantages: Natural Language Processing’s Key Drawbacks
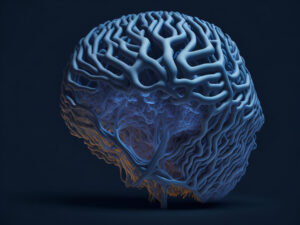
NLP has been prone to hallucination and mix ups, leading to compromised quality.
Natural language processing and the large language models it powers still face a variety of challenges that hold back the technology from achieving its full potential. The primary challenge faced by NLP encompasses the complexity of human language itself and the various nuances that pervade its expanse. Most languages have words that are bound to have contextual meanings, along with numerous occurrences of ambiguity. These features are just two of several linguistic attributes that complicate the AI protocol’s understanding of human language. In addition, since several NLP programs power generative AI, any errors in understanding will inevitably result in erroneous production of AI-generated content, ultimately leading to lost time and resources. Scientists have been working on various methods to solve the problems associated with ambiguity, subjectivity, and colloquialisms that might be endemic to native speakers and specific dialects of a particular language.
Current NLP algorithms might also be limited in the extent of their data since there are several different languages and their respective dialects. Often, the most challenging aspect of training language models involves the highly unique attributes of language such as slang, context, and insinuation. Machines often find it difficult to process contextual meanings and implied information that might otherwise be understood rather instantly by a human. Also, most current NLP models for machine learning are restricted to English. While chatbots such as Google Bard, ChatGPT, and Anthropic’s Claude are also gradually featuring more languages, it will be a while until NLP models and LLMs become highly proficient in languages other than English, even in aspects of colloquialisms and slang.
Addressing NLP’s Drawbacks and Other AI Disadvantages
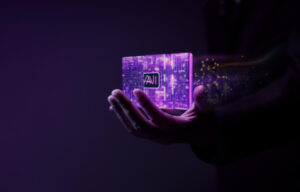
Language models will have to be built on more robust systems to counter the prevailing challenges faced by NLP.
Addressing the challenges faced by natural language processing algorithms will need to be multifaceted, involving both technical and linguistic components that function in tandem. Among the biggest challenges are biased responses that might result in erroneous results. Tackling this will require a comprehensive dataset that encompasses the various facets of language and dynamic training models. By instituting human testing protocols that put the machine through numerous scenarios to judge its database, this can be mitigated. The technical aspects would involve building robust deep-learning protocols to support linguistic proficiency. In addition, it would also require datasets free of noise and irrelevant data that might cloud the machine’s judgment. Word distribution and embedding will also have to be addressed sufficiently to ensure all text generated is coherent and to ensure quality AI writing throughout.
Another important method that can help weed out certain issues in NLP algorithms and language models involves the deployment of sentiment analysis, which can, over time, allow LLMs to understand the sentiment expressed in a given statement. Several LLMs and chatbots, such as Claude 2 and the friendliness-focused Inflection 1 and Inflection 2, utilize advanced sentiment analysis techniques to provide high-quality and safe responses to users. Lastly, as technologies improve and artificial intelligence becomes more ubiquitous in applications across the board, the quality of responses shall improve proportionally. Similarly, feedback systems to incorporate suggestions from users might also help.
The Prospects for Natural Language Processing AI
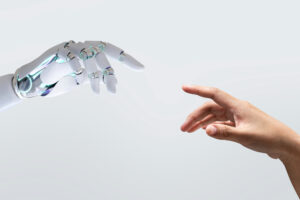
Despite current drawbacks, NLP and LLMs continue to remain the most cutting-edge technologies of the present age.
While there remain several challenges faced by NLP, the technology has become a significant part of modern workflows and customer-facing applications. The past year-and-a-half has been extremely significant in the generation of generative and predictive AI protocols, which have been boosted by natural language processing. Moreover, NLP has also paved the way for advanced AI algorithms such as generative adversarial networks and image generation platforms. The power of machines to understand human language is set to usher in a new era for computing and artificial intelligence shortly.
FAQs
1. Why is NLP limited in its scope?
Natural language processing algorithms often find it difficult to encompass idiosyncratic aspects of slang, contextual meaning, and nuance. This makes their application limited in certain aspects.
2. What is the purpose of language models?
Language models have a variety of applications. However, the core of these remains centered around achieving a better understanding of human language to help computers provide better outputs.
3. What are the core challenges of NLP algorithms?
The core challenges of NLP include lack of data, hallucinations, and AI bias.