Google DeepMind—Google’s premium AI research laboratory—has made breakthroughs in materials science with its latest GNoME models. GNoME, an abbreviation for Graph Networks for Materials Exploration, is a set of graph neural networks (GNN) that has successfully discovered over 2.2 million new crystals, which could revolutionize numerous sectors such as semiconductor manufacturing, energy infrastructure, and more. Of the 2.2 million crystals discovered, DeepMind claims about 380,000 are the most stable among the lot. These developments are crucial since humans are constantly on the lookout for new crystals and stable materials for a variety of tasks, which include the creation of efficient batteries, enhanced computer chips, and electrically conductive lightweight materials.
The process of material discovery in the manual domain is a long, drawn-out, and arduous process that involves several steps of trial and error, often producing only limited rates of success. However, the integration of machine learning protocols and the innate ability of these technologies to identify and extrapolate based on recurring patterns make the process simpler and possibly more cost-effective. The utilization of new materials can result in the development of cutting-edge technologies capable of powering humanity’s collective future while also addressing key challenges faced by current civilization.
What Are Graph Neural Networks, and How Does GNoME Function?
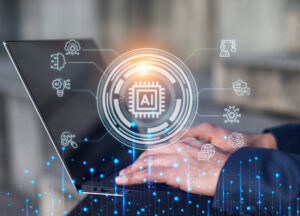
Graph neural networks can be very useful in physical sciences.
Graph neural networks, like Google GNoME, function on data and underlying information primarily based on graphical representations. It is an intuitive method of training language models with highly specific deep learning protocols, which can produce efficient predictive AI systems. Besides materials science, GNNs are also deployed in AI applications that might be developing natural language processors, social media applications, and other scientific domains such as applied physics and molecular biology. GNNs have several different variations that can be augmented and deployed based on the varying requirements of the application. Google GNoME primarily focused on learning the specifics of crystals and how different molecules form varied structures. GNoME was especially efficient since the atomic bonds in the molecules and their subsequent crystal lattices could be represented as graphical data.
GNoME was further trained on aspects of materials science that are used to compute a given structure’s stability using established protocols in both physics and chemistry. The data primarily consisted of information that was publicly available through The Materials Project, which makes available information about various novel compounds and crystals to researchers and independent organizations for free. GNoME also comprises a generative AI component since it extrapolates based on the data it has been trained on to predict several iterations of stable compounds and their resulting structures. Needless to say, this has far-reaching implications for the scientific and industrial communities as a whole, an aspect that will be explored in the forthcoming section.
Why Are Google GNoME and DeepMind’s Discoveries Significant for Materials Science?
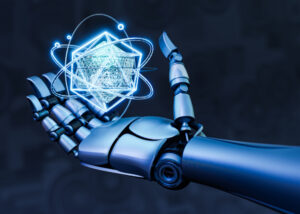
Google GNoME’s discoveries have accelerated the detection of new materials exponentially.
Google GNoME made a whopping discovery of over 2.2 million materials that the firm claims to be equivalent to over 800 years’ worth of human knowledge. Of this vast number, 380,000 are said to be stable. More interestingly, external researchers and laboratories were able to successfully synthesize 736 of these crystalline structures independently, making Google GNoME’s discovery all the more credible. As artificial intelligence is gaining firmer footing in the STEM disciplines, this further bolsters the domain’s credibility in assisting human innovation. Researchers at the Lawrence Berkeley National Laboratory also published a paper detailing their findings that further extend support for GNoME’s AI-based structural predictions that have helped create several stable crystalline materials.
Presently, crystal structures remain crucial in industries such as renewable energy and computing since they form the base of solar cells and computer chips. Stable structures can help create sustainable and cost-effective materials that can then be used to power human technology. It is known that materials like graphene have the potential to revolutionize the way electricity is transmitted. Similarly, the discovery of new crystal lattices and potential materials can further help proliferate advanced technology. Google DeepMind’s GNoME also has implications for AI-generated content as well as AI and ML as a whole, since the industry is a major consumer of advanced chips and requires vast amounts of electricity to function. Given that AI also has a considerable impact on the environment, novel material that can enhance the judicious use of power can help improve artificial intelligence’s present environmental quotient. Further, DeepMind will be contributing the 380,000 stable structures discovered by GNoME to the Materials Project to continue supporting independent research and the exploration of new compounds.
The Future of AI Science and the Impact of Google GNoME
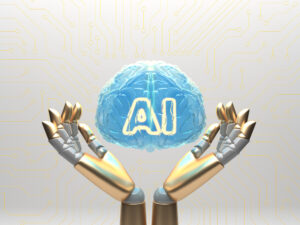
AI models like GNoME have huge potential for future scientific advancement.
AI has been touted as a key factor in the future of science since the technology essentially takes away the burden of repetitive and cognitively laborious tasks and instead allows its human operators to stay focused on more creative aspects of their tasks. That being said, AI has great potential for sorting through data and information, allowing big data to become an integral part of the way current scientists approach their evidence-based practices. By streamlining workflows and enhancing the efficiency of each research project, AI can help build sustainable futures for humanity at large. However, GNoME is still a limited model and will require consistent upgrades to keep pace with growing demand. Moreover, AI in itself is also prone to a few pitfalls, such as bias and hallucination, which will need to be addressed conclusively before adopting these technologies into high-stakes scientific applications on a large scale.
FAQs
1. What does Google GNoME stand for?
Google GNoME is short for Graph Networks for Materials Exploration, a new Graph Neural Network-based model that can predict and discover new crystal structures.
2. How many materials did Google GNoME discover?
Google GNoME discovered over 2.2 million new crystal structures, of which 380,000 are said to be stable. Scientists from independent research facilities synthesized 736 of these crystalline materials, further adding weight to GNoME’s discoveries.
3. Who created Google GNoME?
GNoME was created by Google DeepMind, which happens to be Google’s premier AI research laboratory and is known for the creation of several advanced AI protocols.